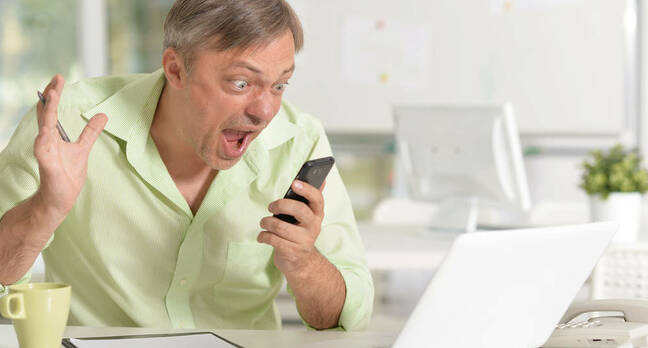
A thousand spoken words is worth a picture
Machine learning researchers are on a mission to make machines understand speech directly from audio input, like humans do.
At the Neural Information Processing Systems conference this week, researchers from Massachusetts Institute of Technology (MIT) demonstrated a new way to train computers to recognise speech without translating it to text first.
The presentation was based on a paper written by researchers working at MIT's Computer Science and Artificial Intelligence Laboratory (CSAIL).
The rise of interest in deep learning has accelerated the performance of computer speech recognition. Computers can achieve lower word error rates than professional transcriptionists, but it requires intense training.
Researchers have to label audio input with transcriptions containing the right text in order for the machines to match sounds to words. It requires careful data collection, and thousands of languages are still unsupported by speech recognition systems.
It's a problem that needs tackling if the technology is to be beneficial for society, said Jim Glass, coauthor of the paper and leader of the Spoken Language Systems Group at CSAIL.
"Big advances have been made – Siri, Google – but it's expensive to get those annotations, and people have thus focused on, really, the major languages of the world. There are 7,000 languages, and I think less than 2 percent have ASR [automatic speech recognition] capability, and probably nothing is going to be done to address the others.
"So if you're trying to think about how technology can be beneficial for society at large, it's interesting to think about what we need to do to change the current situation," Glass said.
The ultimate goal would be to create machines that could understand the complexities of language and meanings of words without having to process speech into text first – a process that requires something called "unsupervised learning."
Researchers from MIT have attempted to do this by mapping speech to images instead of text.
The idea is that if words can be grouped together as a set of related images, and these images have associated text, then it should be possible to find a "likely" transcription of the audio without having to undergo rigorous training.
To collect the training dataset, the researchers used the Places205 dataset, which contains over 2.5 million images categorised into 205 different subjects. People working on Human Intelligence Tasks onAmazon Mechanical Turk were paid to describe four random pictures from the Places205 dataset through audio recordings to provide the pictures with captions. (Just in case you're wondering, participants were paid three cents per recording.)
Researchers have collected approximately 120,000 captions from 1,163 unique "turkers" and plan to make their dataset publicly available soon.
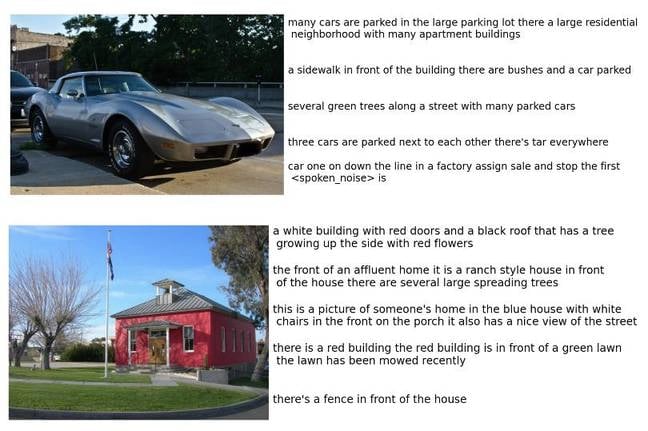
Example of captions for an image in the dataset ... Source: MIT
The model is trained to link words to relevant images, and gives a similarity scoring for each pairing. "In its simplest sense, our model is designed to calculate a similarity score for any given image and caption pair, where the score should be high if the caption is relevant to the image and low otherwise," the paper said.
During the test phase, the researchers fed the network audio recordings describing a picture in its database and it was asked to retrieve ten images that best matched the description. Out of those ten images, it would contain the correct one only 31 per cent of the time.
It's a low score, and the new method is a rudimentary way of giving computers a way of recognising words without any knowledge of text or language, but with improvement it could help speech recognition to adapt to different languages.
Mapping audio input to images isn't immediately useful, but if the system is trained on images that are associated with words from different languages, it may provide a new way to translate speech into other languages.
"The goal of this work is to try to get the machine to learn language more like the way humans do," said Glass. "The current methods that people use to train up speech recognisers are very supervised. You get an utterance, and you're told what's said. And you do this for a large body of data.
"I always emphasize that we're just taking baby steps here and have a long way to go," Glass says. "But it's an encouraging start."
No comments:
Post a Comment